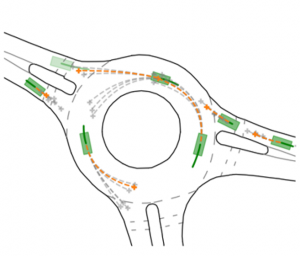
The Centre for Robotics is proud to announce that the PhD Defense of Thomas GILLES will take place on Friday, April 21st at MINES PARIS.
The subject of the work is:
“Smart vehicule trajectory prediction in various autonomous driving scenarios”
This research is the result of a collaboration between our Centre and Huawei France.
The PhD has been directed by Fabien Moutarde, and supervized by Bogdan Stanciulescu.
To attend:
MINES PARIS
60, Saint-Michel boulevard, Paris.
Jury members:
Alexandre ALAHI, Assistant Professor, EPFL
Fawzi NASHASHIBI, Research Director, National Institute for Research in Digital Science and Technology (Inria)
David FILLIAT, Full Professor, ENSTA Paris
Anne SPALANZANI, Full Professor, Université Grenoble Alpes (UGA) / National Institute for Research in Digital Science and Technology (Inria)
John FOLKESSON, Associate Professor, KTH Royal Institute of Technology, Sweden
Dzmitry TSISHKOU, PhD, Huawei Technologies France
Fabien MOUTARDE, Full Professor, MINES PARIS
Bogdan STANCIULESCU, Associate Professor, MINES PARIS
Abstract:
Recent advances in machine learning methods have enabled tremendous progress in autonomous driving, namely through the perception step thanks to deep learning and deep neural networks, combined with all-around progress in sensors, mapping and proprioception techniques. The focus is now therefore shifting towards the next steps in the autonomous pipeline, where prediction plays an important role. Once the surrounding road agents have been detected and tracked, the driving system needs to predict their future trajectory and plan accordingly to have a collision-less course.
This trajectory prediction must follow multiple requirements. First, it should obviously be accurate and trustworthy, so that its output can be reliably used in the following processes. The future can present multiple possibilities, from which it may not always be possible to disambiguate solely based on past historical data. The forecast must therefore be multimodal, by predicting multiple simultaneous probable futures. Since the prediction is to be made on all surrounding agents, and these agents behaviors are very much influenced by their interactions with each other, the model should take these interactions into account, and its multimodal predictions should be coherent with each other. Finally, for safety and reliability, the trajectory prediction should be easy to interpret, extensively evaluated, able to provide confidence evaluates and designed with its final use in the pipeline in mind.
In the first part of this dissertation, after recapitulating existing non-learning methods for trajectory forecasting, we study different existing representations and approaches for learning-based motion forecasting. We then propose to tackle the trajectory prediction problem using probability heatmaps to facilitate multimodality. We design three different ways of generating these heatmaps and evaluate them against each other and the existing state-of-the-art. We also provide a complete sampling method to extract actual trajectories from these heatmaps, and study the pro and cons of these heatmap methods compared to other commonly used frameworks. In the next chapter, we focus on multi-agent prediction, and more specifically consistent scene-level outputs, for these type of heatmap models through sampling and learned post-processing. Finally, we explore different ways of expanding prediction model evaluation by uncertainty assessment, calibration and cross-dataset generalizability analysis.